Alzheimer’s Disease and Related Dementias—A Pressing Challenge for Aging Populations and Healthcare Systems
Dementia is the most prevalent neurodegenerative disorder in aging populations, with Alzheimer’s disease (AD) as its most common and most feared form—robbing individuals of their memories, relationships, and identities. According to the World Health Organization, the global incidence of AD is rising sharply and is expected to reach 152 million cases by 2050. In the United States alone, it is estimated that one person develops AD every 65 seconds on average.
This surge poses a major public health challenge, profoundly affecting individuals’ well-being while placing immense strain on healthcare systems and social infrastructure. In Europe alone, these costs are expected to exceed €250 billion by 2030. In the United States, the financial burden of Alzheimer’s Disease and Related Dementias (ADRD) is equally alarming, with healthcare expenses estimated at $321 billion in 2022, and projections surpassing $1 trillion by 2050.
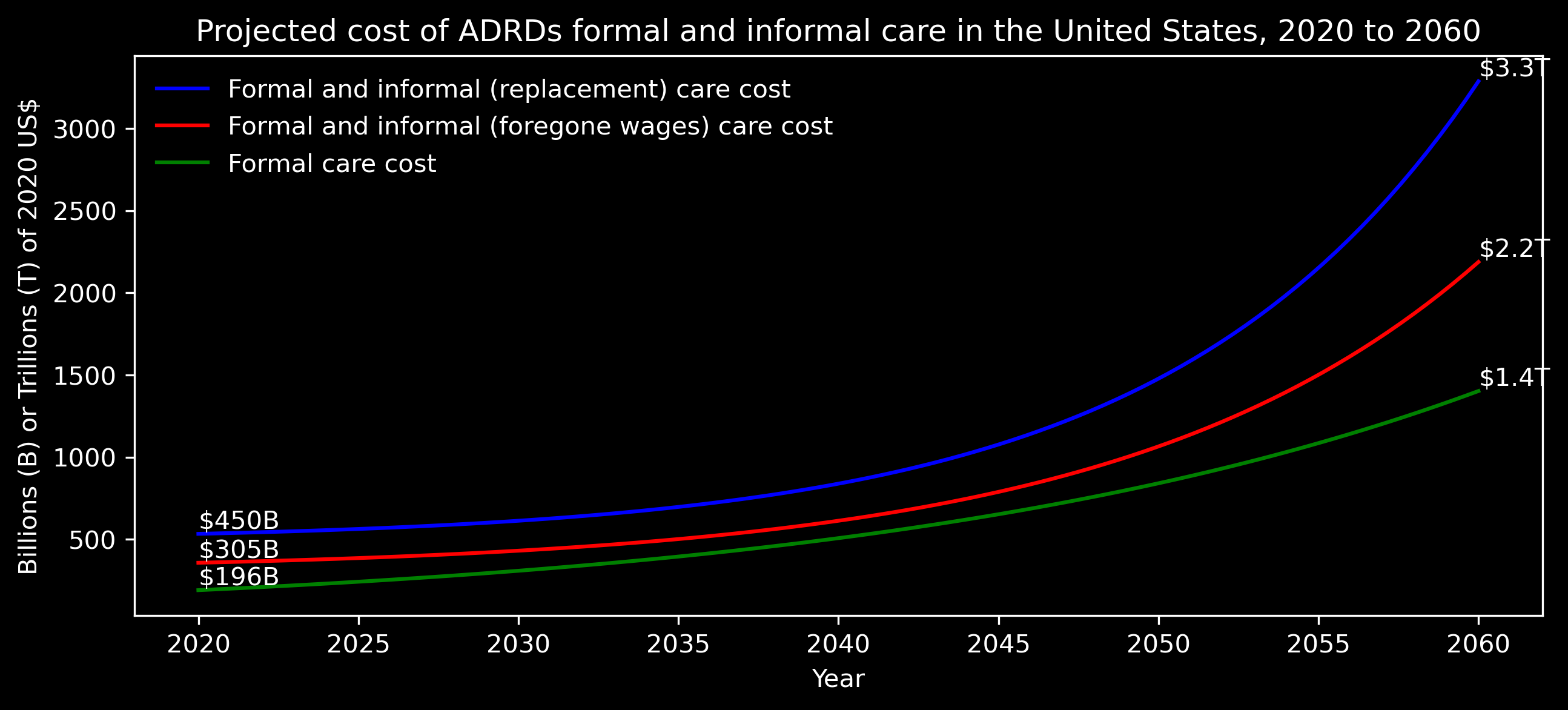
Projected costs of Alzheimer's disease and related dementias (ADRD) in the United States for formal and informal care between 2020 and 2060, based on data from Nandi, et al. (2024). Cost of care for Alzheimer’s disease and related dementias in the United States: 2016 to 2060. npj Aging, 10(1),13.
Why is Early Detection of Alzheimer’s Disease and Related Dementias Critical?
Dementia is a progressive neurodegenerative disorder characterized by cognitive decline and functional impairment, posing significant challenges in clinical management. As an irreversible condition, dementia progresses through distinct stages, from preclinical to mild, moderate, and severe [1]. Research indicates that the build-up of pathology in AD begins decades before clinical symptoms manifest [2]. Therefore, early cognitive assessment and diagnosis are crucial for delaying or potentially preventing the irreversible progression of AD. Empirical data suggests that approximately 40% of Alzheimer’s risk factors are modifiable in the early stages [3], offering a critical window for interventions aimed at preventing or slowing cognitive decline. Moreover, the emergence of disease-modifying treatments for AD underscores the critical importance of early detection. These therapies are most effective during the disease’s earliest stages, making timely identification essential for optimal outcomes.
Despite this, Alzheimer’s is not routinely screened for in clinical practice. Diagnosis typically occurs only when patients report cognitive concerns or when impairment significantly disrupts daily life—often missing the opportunity for early intervention.
The Need for Scalable, Accessible Screening Tools
Advancements in accessible, scalable screening tools are essential for early Alzheimer’s detection and monitoring its progression. Effective screening tools will enable timely interventions and decentralized clinical trials. Current screening methods—including cognitive tests, MRI, and cerebrospinal fluid (CSF) analysis—are impractical for frequent, large-scale population screening, being expensive, time consuming and/or invasive, leaving over 55% of dementia cases undiagnosed in the US [4]. This challenge has driven efforts to develop affordable, non-invasive alternatives, paving the way for more effective and widely accessible solutions in both clinical settings and population screening.
Blood-based screening is emerging as a lower cost diagnostic tool and is expected to approach clinical application in the coming years [5]. However, research to date indicates that blood-based biomarkers do not differentiate between the clinical stages of Alzheimer’s disease [6]. As a result, while blood-based diagnostics are becoming more sensitive and reliable, there will still be a critical need for complementary cognitive and clinical assessments. Moreover, blood tests are still invasive, require in-person visits, and typically take several days to weeks for results.
In contrast, speech-based diagnostic assessments offer a non-invasive, remote alternative with real-time, automated results—providing a significant advantage for large-scale, decentralized screening and continuous monitoring.
How AI-Powered Digital Biomarkers from Speech and Language Can Democratize Screening and Enable Early Detection
Numerous studies have demonstrated that connected speech in individuals with neurodegenerative diseases deviates significantly from that of healthy aging controls [7]. Speech fluency is disrupted, as reflected in slower speech rates, prolonged pauses, and a higher occurrence of hesitation markers. Syntactic complexity is also reduced, often manifesting in simpler sentence structures. Furthermore, lexical diversity, sophistication and richness are diminished, characterized by a greater reliance on generic terms, less precise word choices, and lower type-token ratios.
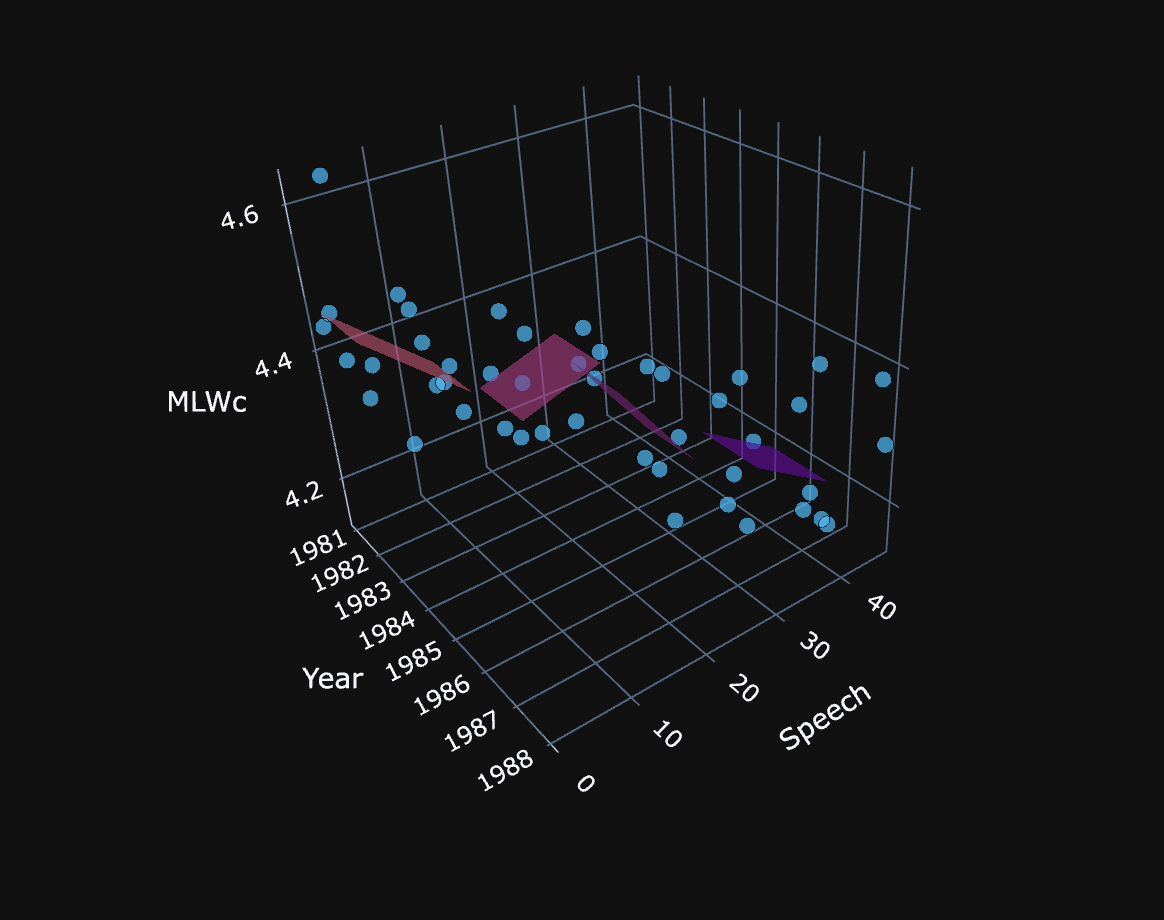
The scatter plot shows a decline in lexical sophistication, measured by the Mean Length of Words in Characters (MLWc), in President Ronald Reagan’s unscripted (spontaneous) speech during press conferences from 1981 to 1988. Each point represents an individual speech sample, while the regression surface highlights a pattern of plateaus followed by sharp declines. President Reagan, who served as the 40th President of the United States from 1981 to 1989, was diagnosed with Alzheimer’s disease in 1994, six years after leaving office.
Recent advances in Natural Language Processing and Machine Learning have enabled the identification of intricate statistical patterns across multiple linguistic and acoustic dimensions, facilitating the extraction of quantifiable speech metrics. These developments mark a paradigm shift in ADRD diagnostics [8]. By providing rapid, reproducible, and objective assessments, these technologies are increasingly recognized for their potential to enhance our understanding of language impairments associated with neurodegenerative processes in the brain [9].
One of the most promising aspects of these advancements is their potential to democratize screening for ADRD. Unlike traditional diagnostic methods for neurodegenerative disorders—which are often time-consuming, expensive, and invasive—AI-powered speech and language analytics provide a non-invasive, accessible, and cost-effective alternative. Leveraging the widespread availability of smartphones, voice assistants, and other digital devices, individuals can benefit from continuous, real-time speech monitoring through active or passive data collection. This accessibility could enable large-scale screening and early detection, particularly for populations with limited access to specialized medical facilities.
Early Detection of ADRD
The ability to monitor subtle speech changes over time offers an unprecedented opportunity for the early detection of ADRD. AI-powered digital biomarkers can identify deviations from normal speech patterns, potentially signaling the onset of cognitive decline long before traditional clinical symptoms emerge. Research has shown that changes in language usage and speech patterns can be detected in connected speech well before a clinical diagnosis of AD is made [10]. This proactive approach could facilitate earlier interventions, allowing patients and their families to seek treatment sooner. Such timely action has the potential to slow disease progression and significantly improve quality of life.
Monitoring Disease Progression
AI-powered speech biomarkers also hold promise for monitoring disease progression. By tracking speech changes over time, clinicians can gain valuable insights into how quickly a patient’s condition is advancing. This information can help create personalized care plans tailored to the individual’s specific needs, ensuring that interventions are timely and targeted.
Measuring Treatment Response
Another key benefit of AI-powered speech analysis is its potential to assist in measuring treatment response. As new therapies emerge, it is essential to track whether a patient is responding positively to treatment. Traditional clinical assessments can be subjective and slow, but speech-based biomarkers offer an immediate, objective way to assess the effectiveness of interventions. This data can inform clinical decisions, enabling doctors to adjust treatments as needed for better outcomes.
The Need for Standardization in Selection and Measurement of AI-Driven Digital Speech Biomarkers
As AI-driven speech biomarkers for neurodegenerative diseases continue to advance, one of the primary challenges is ensuring that the features being measured are both reliable and clinically meaningful. Before we can identify the most predictive features of speech—such as pause length, speech rate, syntactic complexity, and lexical diversity—we must first establish consistent, standardized methods for measuring these features accurately. Once we have robust, standardized measurements, the next step is to determine which of these features are most predictive of cognitive decline, disease progression, and treatment response. This process of feature identification is essential for creating reliable AI models that can be trusted in clinical applications, making it crucial to systematically evaluate which features truly contribute to predictive accuracy.
One of the key catalysts driving progress in this field has been the establishment of international shared task competitions. These competitions offer a unique platform for researchers and organizations to benchmark their algorithms using a common dataset, fostering collaboration and accelerating innovation. The goal of these public challenges is two-fold: (1) to spur translation of new featurization and modeling techniques into practical applications, and (2) to establish a clear definition of state-of-the-art model performance.
In Alzheimer’s disease research, the ADReSS 2020 and ADReSSo 2021 challenges have been instrumental in driving progress. The ADReSSo 2021 (Alzheimer’s Dementia Recognition through Spontaneous Speech) challenge required participants to build models directly from speech, bypassing manual transcription by utilizing automatic speech recognition (ASR) systems. The ADReSSo dataset was carefully designed to minimize common biases in Alzheimer’s detection, such as variations in audio quality, and imbalances in gender and age distributions. A core objective was to develop a model capable of distinguishing between speech from individuals with Alzheimer’s disease (AD) and non-AD participants. Researchers could either use raw acoustic features or employ ASR to generate transcriptions and extract linguistic features. The baseline results revealed that linguistic features significantly outperformed acoustic features, achieving an accuracy of 77.46% compared to 64.79% for acoustic features. While acoustic features remain relevant, these findings emphasize the need for more sophisticated feature selection and modeling approaches to improve predictive accuracy and clinical applicability.
In this ADReSSo 2021 Challenge, our Machine Learning models have already achieved 83.1% accuracy in detecting Alzheimer’s dementia from spontaneous speech in that ADReSSo 2021 Challenge. For more details, check out our paper here.
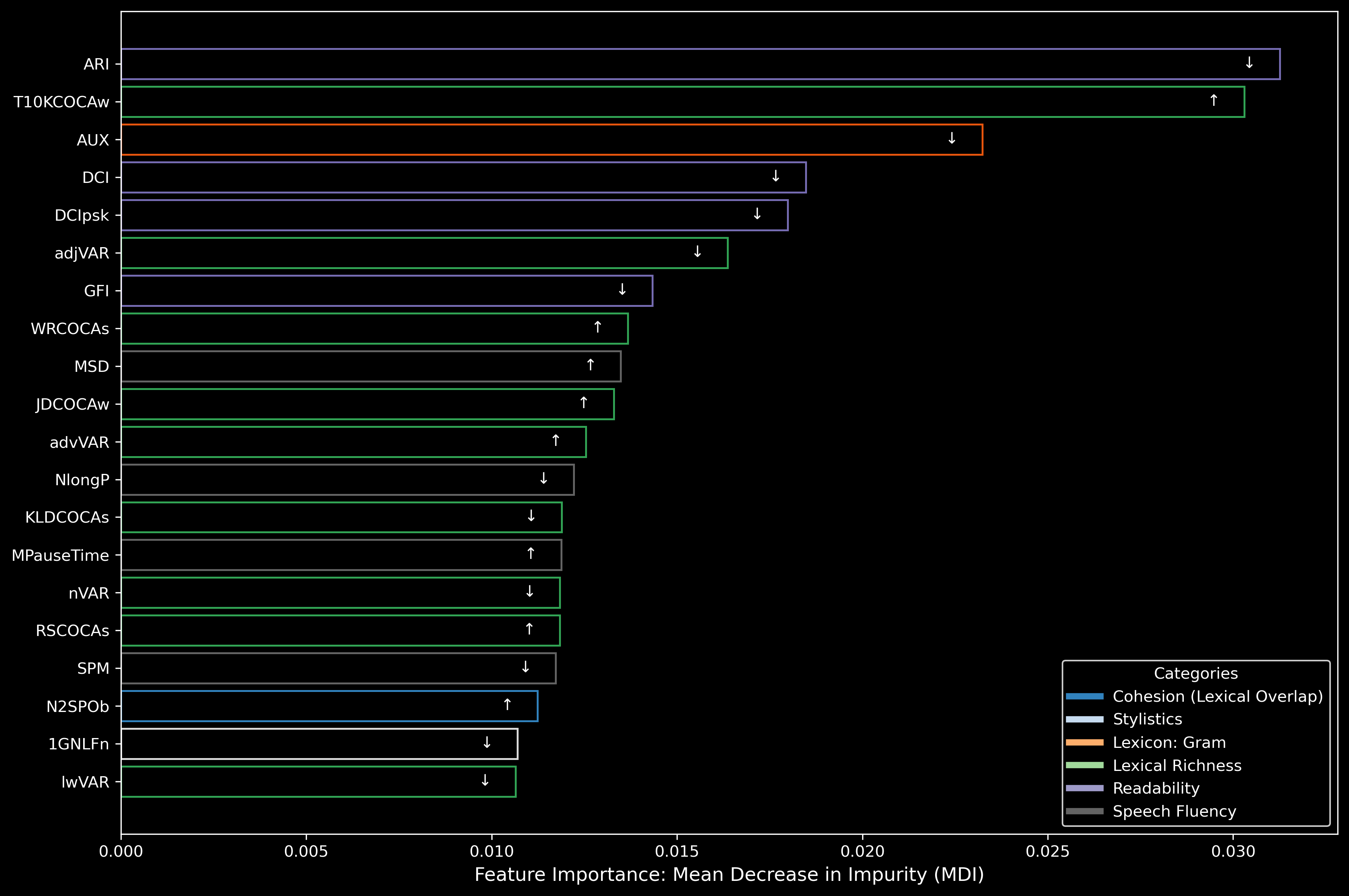
Feature ablation study results using CYMO-extracted features from the ADReSSo 2021 Challenge training dataset for automatic Alzheimer’s disease (AD) detection from spontaneous speech. Feature importance is quantified using the Mean Decrease in Impurity (MDI) metric, where longer bars indicate greater influence on model decisions. Arrows (↑ and ↓) denote whether the average feature value is higher in AD or control groups .
Why AI-Driven Digital Biomarkers by Exaia Technologies?
At Exaia, we are at the forefront of developing AI-driven digital biomarkers derived from spontaneous speech and language, offering a scalable, non-invasive, and cost-effective solution for screening neurodegenerative diseases, monitoring disease progression, and assessing treatment response. CYMO is central to this approach. This next-generation software tool is designed to extract high-resolution measurements of digital biomarkers across all relevant dimensions, including syntactic complexity, lexical diversity/richness/sophistication, cohesion, stylistics, emotion/sentiment, speech fluency, readability metrics, and more.
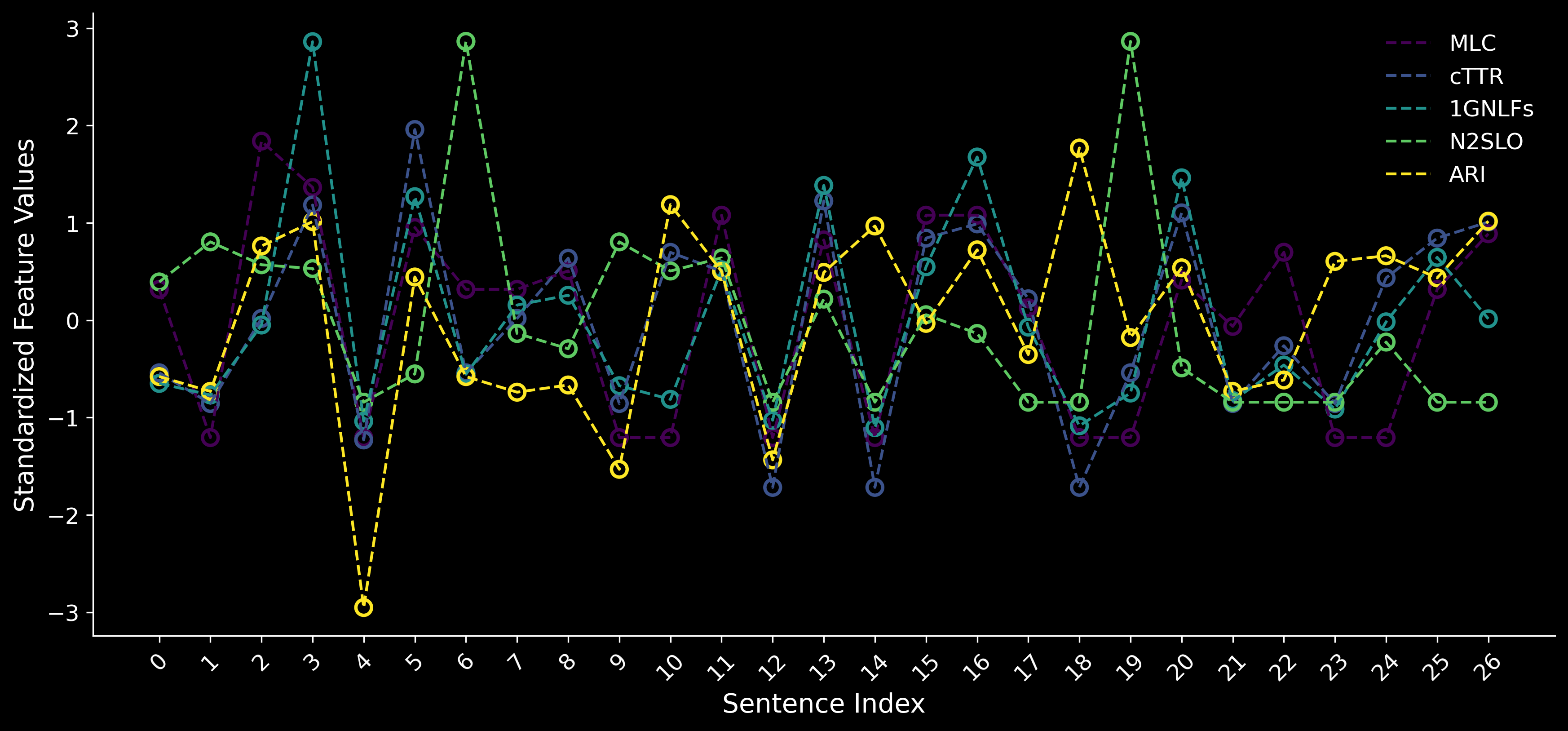
High-resolution measurements of five selected expert-engineered features obtained by CYMO. The standardized feature values are plotted across sentence indices, capturing their variation within a speech sample from the dataset used in The ADReSSo Challenge: Alzheimer's Dementia Recognition through Spontaneous Speech.
It’s time to move beyond ad-hoc selections of biomarkers. We need an all-in-one solution like CYMO, which offers precise measurement resolution based on sophisticated software engineering for feature selection and measurement. This enables the establishment of reliable biomarkers, applicable in real-world scenarios for training robust, generalizable machine learning models.
By harnessing the power of AI and digital speech biomarkers, we can bridge the diagnostic gap, democratizing early detection and giving millions the chance for earlier intervention, improved outcomes, and better quality of life 🌟.
Try out the CYMO demo today and experience the future of digital biomarkers firsthand!
References
[1] Vermunt, L., Sikkes, S. A., Van Den Hout, A., Handels, R., Bos, I., Van Der Flier, W. M., Kern, S., Ousset, P. J., Maruff, P., & Skoog, I. (2019). Duration of preclinical, prodromal, and dementia stages of Alzheimer’s disease in relation to age, sex, and APOE genotype. Alzheimer’s & Dementia, 15(7), 888–898. Back to text
[2] Ritchie, K., Carrère, I., Su, L., O’Brien, J. T., Lovestone, S., Wells, K., et al. (2017). The midlife cognitive profiles of adults at high risk of late-onset Alzheimer’s disease: The PREVENT study. Alzheimer’s & Dementia, 13(10), 1089–1097. Back to text
[3] Livingston, G., Huntley, J., Sommerlad, A., Ames, D., Ballard, C., Banerjee, S., et al. (2020). Dementia prevention, intervention, and care: 2020 report of the Lancet Commission. The Lancet, 396(10248). Back to text
[4] Prince, M., Comas-Herrera, A., Knapp, M., et al. (2016). World Alzheimer Report 2016: Improving healthcare for people living with dementia: Coverage, quality, and costs now and in the future. Alzheimer’s Disease International. Back to text
[5] Teunissen, C. E., Verberk, I. M. W., Thijssen, E. H., et al. (2022). Blood-based biomarkers for Alzheimer’s disease: Towards clinical implementation. The Lancet Neurology, 21, 66–77. Back to text
[6] Tosun, D., Veitch, D., Aisen, P., et al. (2021). Detection of β-amyloid positivity in Alzheimer’s Disease Neuroimaging Initiative participants with demographics, cognition, MRI, and plasma biomarkers. Brain Communications, 3, fcab008. Back to text
[7] Boschi, V., Catricala, E., Consonni, M., Chesi, C., Moro, A., & Cappa, S. F. (2017). Connected speech in neurodegenerative language disorders: a review. Frontiers in psychology, 8, 269. Back to text
[8] Clarke, N., Foltz, P., & Garrard, P. (2020). How to do things with (thousands of) words: Computational approaches to discourse analysis in Alzheimer’s disease. Cortex, 129, 446-463. Back to text
[9] de la Fuente Garcia, S., Ritchie, C., & Luz, S. (2020). Artificial intelligence, speech, and language processing approaches to monitoring Alzheimer’s disease: A systematic review. Journal of Alzheimer’s Disease, 78(4), 1547–1574. Back to text
[10] Karr, J. E., Graham, R. B., Hofer, S. M., and Muniz-Terrera, G. (2018). When does cognitive decline begin? A systematic review of change point studies on accelerated decline in cognitive and neurological outcomes preceding mild cognitive impairment, dementia, and death. Psychol. Aging 33, 195–218.. Back to text